Information gain ratio
|
Read other articles:
Keuskupan KumboDioecesis KumboensisKatolik LokasiNegaraKamerunWilayahDivisi Bui dan Donga-Mantung.Provinsi gerejawiBamendaDekanat6StatistikLuas8.600 km2 (3.300 sq mi)Populasi- Total- Katolik(per 2011)789.000202,543 (25.7%)Paroki27Imam83InformasiDenominasiKatolik RomaRitusRitus LatinPendirian18 Maret 1982KatedralGereja Katedral Santa Teresa dari Kanak-Kanak YesusKepemimpinan kiniPausFransiskusUskupGeorge NkuoUskup agungCornelius Fontem EsuaVikaris jenderalRol...

Muhidin M. Said Anggota Dewan Perwakilan RakyatPetahanaMulai menjabat 1 Oktober 2004Daerah pemilihanSulawesi TengahAnggota Majelis Permusyawaratan RakyatMasa jabatan1992–2004Grup parlemenUtusan Daerah Sulawesi Tengah Informasi pribadiLahirMuhidin Mohamad Said7 Oktober 1950 (umur 73)Soppeng, Sulawesi, IndonesiaPartai politikGolkarSuami/istriSri SulistiatiAnak5Alma materUniversitas TadulakoJakarta Institute of Management StudiesPekerjaanPengusahaPolitikusSunting kotak info • L...

MualLukisan tahun 1681 yang menggambarkan seseorang muntahICD-10R11.0ICD-9787.03MedlinePlus003117MeSHD009325 Mual atau loya adalah perasaan tidak menyenangkan yang ada sebelum muntah.[1] Ini biasa disertai berkeringat, bertambahnya air liur, dan kontraksi ritmis otot-otot dinding perut.[1] Dalam sumber lain mual adalah suatu kondisi di mana seseorang mempunyai perasaan yang menekan dan tidak nyaman sebelum muntah, tetapi tidak selalu menyebabkan muntah.[2] Mual setelah...

Pour les articles homonymes, voir Lesur. Annie Lesur Fonctions Secrétaire d'État chargée de l'Enseignement préscolaire 8 juin 1974 – 12 janvier 1976(1 an, 7 mois et 4 jours) Président Valéry Giscard d'Estaing Gouvernement Chirac I Prédécesseur Poste créé Successeur Poste supprimé Biographie Nom de naissance Anne-Marie Alice Renée Charpin Date de naissance 28 mars 1926 Lieu de naissance Saint-Honoré-les-Bains (Nièvre) Date de décès 8 septembre 2021 (à 95 ...

Published theory of Samuel P. Huntington about cultural geography The Clash of Civilizations and the Remaking of World Order AuthorSamuel P. HuntingtonCountryUnited StatesLanguageEnglishPublisherSimon & SchusterPublication date1996ISBN978-0-684-84441-1 The Clash of Civilizations is a thesis that people's cultural and religious identities will be the primary source of conflict in the post–Cold War world.[1][2][3][4][5] The American political scient...

American politician (1936–2013) This article needs additional citations for verification. Please help improve this article by adding citations to reliable sources. Unsourced material may be challenged and removed.Find sources: William J. Coyne – news · newspapers · books · scholar · JSTOR (November 2020) (Learn how and when to remove this template message) William J. CoyneMember of the U.S. House of Representativesfrom Pennsylvania's 14th...

Antiretroviral medication AZT redirects here. For other uses, see AZT (disambiguation). ZidovudineClinical dataTrade namesRetrovir, othersAHFS/Drugs.comMonographMedlinePlusa687007License data EU EMA: by INN US DailyMed: Zidovudine Pregnancycategory AU: B3 Routes ofadministrationBy mouth, intravenous, rectal suppositoryATC codeJ05AF01 (WHO) Legal statusLegal status UK: POM (Prescription only)[2] US: WARNING[1]Rx-only[3] EU:...

Dieffenbach-au-Valcomune Dieffenbach-au-Val – Veduta LocalizzazioneStato Francia RegioneGrand Est Dipartimento Basso Reno ArrondissementSélestat-Erstein CantoneMutzig TerritorioCoordinate48°19′N 7°20′E / 48.316667°N 7.333333°E48.316667; 7.333333 (Dieffenbach-au-Val)Coordinate: 48°19′N 7°20′E / 48.316667°N 7.333333°E48.316667; 7.333333 (Dieffenbach-au-Val) Superficie2,96 km² Abitanti638[1] (2009) Densità215,54 ab./...

「俄亥俄」重定向至此。关于其他用法,请见「俄亥俄 (消歧义)」。 俄亥俄州 美國联邦州State of Ohio 州旗州徽綽號:七葉果之州地图中高亮部分为俄亥俄州坐标:38°27'N-41°58'N, 80°32'W-84°49'W国家 美國加入聯邦1803年3月1日,在1953年8月7日追溯頒定(第17个加入联邦)首府哥倫布(及最大城市)政府 • 州长(英语:List of Governors of {{{Name}}}]]) •&...

Pour les articles homonymes, voir Tatum. Channing Tatum Channing Tatum au Comic-Con de San Diego, en 2017. Données clés Naissance 26 avril 1980 (44 ans) Nationalité Américaine Profession Acteur, danseur, mannequin, producteur, réalisateur Films notables Sexy Dance21Magic MikeJe te prometsWhite House Down modifier Channing Tatum est un acteur, danseur, mannequin, réalisateur et producteur de cinéma américain, né le 26 avril 1980 à Cullman (Alabama). Après avoir été révélé...

密西西比州 哥伦布城市綽號:Possum Town哥伦布位于密西西比州的位置坐标:33°30′06″N 88°24′54″W / 33.501666666667°N 88.415°W / 33.501666666667; -88.415国家 美國州密西西比州县朗兹县始建于1821年政府 • 市长罗伯特·史密斯 (民主党)面积 • 总计22.3 平方英里(57.8 平方公里) • 陸地21.4 平方英里(55.5 平方公里) • ...

Clothing company For other uses, see White Stag. White StagCompany typeSubsidiaryIndustryApparelFounded1907HeadquartersUnited States ProductsSport clothing, sportswearParentWal-Mart Stores, Inc. White Stag is an in-store brand of women's clothing and accessories sold by Walmart. Founded as a skiwear manufacturer in Portland, Oregon, the company was purchased by the Warnaco Group in 1966, which in turn sold the brand to Wal-Mart in 2003. Company origins Tag from a Hirsch-Weis sleeping bag...

United States Department of Education research branch Mark Schneider, current director The Institute of Education Sciences (IES) is the independent, non-partisan statistics, research, and evaluation arm of the U.S. Department of Education. IES' stated mission is to provide scientific evidence on which to ground education practice and policy and to share this information in formats that are useful and accessible to educators, parents, policymakers, researchers, and the public.[1] It wa...

Berikut adalah daftar munisipalitas di provinsi Girona, di wilayah otonomi Catalunya Spanyol Peta munisipalitas di Provinsi Girona Nama Populasi (2007) Agullana 756 Aiguaviva 594 Albanyà 137 Albons 620 Alp 1.467 Amer 2.258 Anglès 5.211 Arbúcies 6.232 Argelaguer 418 L'Armentera 770 Avinyonet de Puigventós 1.241 Banyoles 17.309 Bàscara 900 Begur 4.076 Bellcaire d'Empordà 672 Besalú 2.257 Bescanó 3.859 Beuda 164 La Bisbal d'Empordà 9.261 Biure 236 Blanes 37.819 Boadella d'Empordà 230 B...

Pesta Olahraga Asia Tenggara 1997Tuan rumahJakarta IndonesiaJumlah negara10Jumlah atlet6007 (termasuk ofisial)Jumlah disiplin440 dari 34 cabang olahragaUpacara pembukaan11 Oktober 1997Upacara penutupan19 Oktober 1997Dibuka olehSoehartoPresiden Republik IndonesiaDitutup olehSoehartoPresiden Republik IndonesiaTempat utamaStadion SenayanSitus webPesta Olahraga Asia Tenggara 1997← Chiangmai 1995 Bandar Seri Begawan 1999 → Pesta Olahraga Negara-Negara Asia Tenggara 1997 (bahasa In...

2001 greatest hits album by the Backstreet Boys The Hits – Chapter OneGreatest hits album by Backstreet BoysReleasedOctober 23, 2001[1]RecordedOctober 1994 – April 2001Studio Parc (Orlando, Florida, U.S.) Platinum Post (Orlando, Florida, U.S.) Cheiron (Stockholm, Sweden) Polar (Stockholm, Sweden) Matiz (Hamburg, Germany) Powerplay (Zurich, Switzerland) Battery (New York City, U.S.) Tates Creek High School (Lexington, Kentucky, U.S.) GenrePop[2]Length65:03LabelJiveBacks...

Negara-negara anggota Konvensi Regulasi Perburuan Paus Internasional (biru).[1] Konvensi Regulasi Perburuan Paus Internasional adalah sebuah perjanjian lingkungan hidup internasional yang ditandatangani pada 1946.[2] Konvensi tersebut mengatur kegiatan komersial dan saintifik dari perburuan paus terhadap delapan puluh sembilan negara anggota. Referensi Wikisumber memiliki naskah asli yang berkaitan dengan artikel ini: International Convention for the Regulation of Whaling ^ Me...

Leuilly-sous-Coucy Mairie et église. Administration Pays France Région Hauts-de-France Département Aisne Arrondissement Laon Intercommunalité Communauté de communes Picardie des Châteaux Maire Mandat Christian Zakryenski 2020-2026 Code postal 02380 Code commune 02423 Démographie Gentilé Leuillois(es) Populationmunicipale 436 hab. (2021 ) Densité 34 hab./km2 Géographie Coordonnées 49° 28′ 47″ nord, 3° 21′ 33″ est Altitude Min. 51...
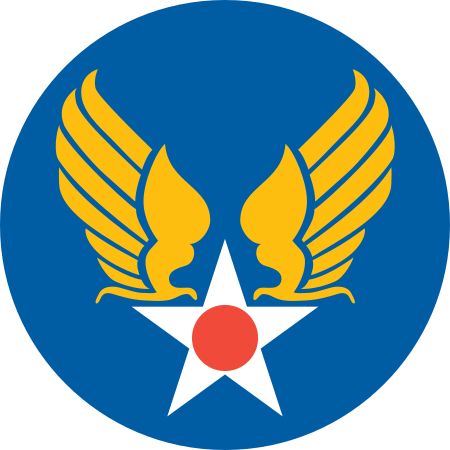
アメリカ陸軍航空軍 創設 1941年6月20日 - 1947年9月17日国籍 アメリカ合衆国軍種 アメリカ陸軍タイプ 陸軍航空隊任務 航空戦上級部隊 アメリカ陸軍主な戦歴 第二次世界大戦表話編歴テンプレートを表示 アメリカ陸軍航空軍(United States Army Air Forces, USAAF)は、かつて存在したアメリカ陸軍の部門。アメリカ空軍の前身である。第二次世界大戦中の1941年に陸軍地上軍と同格�...

City wall in ancient Athens The Piraeus and the Long Walls of Athens Ancient Athens Although long walls were built at several locations in ancient Greece, notably Corinth and Megara,[1] the term Long Walls (Ancient Greek: Μακρὰ Τείχη [makra tei̯kʰɛː]) generally refers to the walls that connected Athens' main city to its ports at Piraeus and Phaleron. Built in several phases, they provided a secure connection to the sea even during times of siege. The walls were ...