K-anonymity
|
Read other articles:

Gabriel Høyland Nazionalità Norvegia Calcio Ruolo Attaccante Carriera Squadre di club1 1970-1986 Bryne? (?) Nazionale 1971 Norvegia U-161 (0)1972-1973 Norvegia U-1911 (3)1974-1977 Norvegia U-214 (0)1974-1982 Norvegia23 (3) Carriera da allenatore 2001 Bryne2009 Bryne 1 I due numeri indicano le presenze e le reti segnate, per le sole partite di campionato.Il simbolo → indica un trasferimento in prestito. Modifica dati su Wikidata · Manuale Gabriel Høyland ...
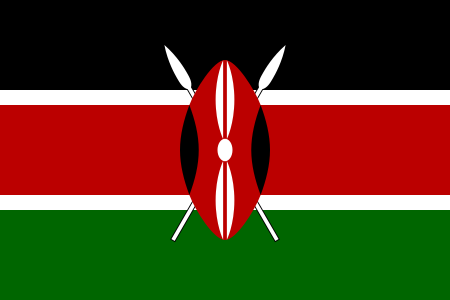
Sporting event delegationKenya at theCommonwealth GamesCGF codeKENCGANational Olympic Committee of KenyaMedalsRanked 8th Gold 85 Silver 75 Bronze 77 Total 237 Commonwealth Games appearances (overview)195419581962196619701974197819821986199019941998200220062010201420182022 Kenya has attended sixteen Commonwealth Games, beginning in 1954 and missing only the 1986 Games. Kenya has won 237 Commonwealth Games medals, mostly in athletics and boxing, with thirty-four coming from a single event, the ...

Adi Parashakti Artikel ini adalah bagian dari seriSakta Ketuhanan tertinggi Adi Parasakti (Mahadewi) Perwujudan Mahadewi Lalita Tripura Sundari Tridewi Saraswati Laksmi Durga Navadurga Mahawidya Kali Sati Parwati Bhairawi Kamakhya (Kubjika) Yogini Tara Uma Camunda Candika Radha Sita Matrika Pustaka suci Weda Upanisad Dewi Sita Tripura Tantra Yoni Kali Kamakalawilasa Kularnawa Mahanirwana Tara Tripura Dewibhagawata Purana Dewi Gita Dewi Mahatmya Lalita Sahasranama Kalika Purana Sutra Parananda...

Dale Van Sickel Dale Harris Van Sickel (Eatonton, Georgia, 29 de noviembre de 1907 - Newport Beach, California, 25 de enero de 1977) fue un jugador estadounidense de fútbol universitario, baloncesto universitario y béisbol universitario durante la años 20, quien después se convirtió en un actor de Hollywood y especialista de cine por más de cuarenta años. Van Sickel jugó al fútbol universitario para la Universidad de la Florida, y su equipo fue reconocido como el primer equipo All-Am...

لمعانٍ أخرى، طالع ميخائيل كيلي (توضيح). هذه المقالة يتيمة إذ تصل إليها مقالات أخرى قليلة جدًا. فضلًا، ساعد بإضافة وصلة إليها في مقالات متعلقة بها. (يوليو 2019) ميخائيل كيلي معلومات شخصية الميلاد 29 مارس 1872 غالواي الوفاة 3 مايو 1923 (51 سنة) كوبلنز مواطنة الولايات...
IseVaro della IseDescrizione generale TipoNave da battaglia ClasseIse CantiereKawasaki, Kōbe Impostazione10 maggio 1915 Varo12 novembre 1916 Completamento15 dicembre 1917 Destino finaleAffondata, 28 luglio 1945 Caratteristiche generaliDislocamentoNormale: 31762 t Pieno carico: 37086 t LunghezzaGlobale: 205,8 m linea di galleggiamento: 195,1 m Larghezza28,7 m Pescaggio8,8 m PropulsioneTurbine Curtiss, 4 assi elica; 24 caldaie Kampon; 45 CV; Carbone 4706 ...
British politician The Right HonourableThe Lord AilwynKCVO KBE PC DLVice-Chamberlain of the HouseholdIn office10 July 1895 – 3 December 1900MonarchQueen VictoriaPrime MinisterThe Marquess of SalisburyPreceded byCharles SpencerSucceeded bySir Alexander Fuller-Acland-Hood, BtPresident of the Board of AgricultureIn office14 March 1905 – 4 December 1905MonarchEdward VIIPrime MinisterArthur BalfourPreceded byThe Earl of OnslowSucceeded byThe Earl Carrington Person...

Peacekeeping unit of the Russian army 15th Separate Guards Motorized Rifle BrigadeRussian: 15-я отдельная гвардейская мотострелковая бригадаShoulder sleeve insigniaActive1 February 2005–PresentCountry RussiaBranch Russian Ground ForcesTypeMechanized infantry (peacekeeping)SizeBrigadePart of2nd Guards Combined Arms Army, Central Military DistrictGarrison/HQRoshchinsky, Volzhsky District, Samara OblastEquipmentTorn-MDM radio intelligenc...

Heppach Oberlaufname: Hörnlesbach Mündung des Heppachs in die Rems Mündung des Heppachs in die Rems Daten Gewässerkennzahl DE: 2383678 Lage Neckarbecken Waiblinger Bucht Remstaltraufbucht Baden-Württemberg Rems-Murr-Kreis Gde. Korb Stadt Weinstadt Flusssystem Rhein Abfluss über Rems → Neckar → Rhein → Nordsee Quelle des Hörnlesbachs:nordöstlich von Korb unterm Hanweiler Sattel48° 50′ 54″ N, 9° 22′...

Revival architectural style This article needs additional citations for verification. Please help improve this article by adding citations to reliable sources. Unsourced material may be challenged and removed.Find sources: Moorish Revival architecture – news · newspapers · books · scholar · JSTOR (December 2007) (Learn how and when to remove this template message) Famed Viječnica in Sarajevo, 1894, building of the National Library of Bosnia and Herzeg...

2014 video gameGuilty Gear XrdTextless cover of the home console versionsDeveloper(s)Arc System WorksPublisher(s)ArcadeJP: SegaPS3, PS4JP: Arc System WorksNA: Aksys GamesEU: Sony Computer Entertainment (Sign)EU: PQube (Revelator, Rev 2)WindowsWW: Arc System WorksDirector(s) Daisuke Ishiwatari Takeshi Yamanaka Designer(s)Daisuke IshiwatariArtist(s)Hidehiko SakamuraWriter(s) Daisuke Ishiwatari Takeshi Yamanaka Yoshito Shoudai Composer(s) Daisuke Ishiwatari Norichika Sato SeriesGuilty GearEngine...

This article has multiple issues. Please help improve it or discuss these issues on the talk page. (Learn how and when to remove these template messages) A major contributor to this article appears to have a close connection with its subject. It may require cleanup to comply with Wikipedia's content policies, particularly neutral point of view. Please discuss further on the talk page. (June 2018) (Learn how and when to remove this template message) This article's lead section may be too short...

El predicador estadounidense Billy Graham en 1966. Un predicador cuáquero y su congregación. Predicador es un término que se aplica a quien pronuncia sermones y homilías, generalmente de contenido teológico. La predicación también se entiende que no se limita a un punto de vista religioso, sino que, en sentido amplio, se extiende a las visiones del mundo moral y social. Los predicadores son comunes en la mayoría de las culturas. Pueden tomar la forma tanto de un ministro cristiano en ...

Swiss female curler and coach Betty BourquinCurler ♀Other namesBetty Bourquin-SteffenTeamCurling clubBasel-Albeina CC, BaselCurling career Member Association SwitzerlandWorld Championshipappearances1 (1979)European Championshipappearances1 (1979) Medal record Curling World Championships 1979 Perth European Championships 1979 Varese Swiss Women's Championship[1] 1979 Betty Bourquin (in marriage also known as Betty Bourquin-Steffen[2]) is a former Swiss fem...

Morena Información personalNombre de nacimiento Margerita Camilleri FenechOtros nombres «El Volcán Mediterráneo»Nacimiento 22 de mayo de 1984Sannat, Malta Nacionalidad MaltesaLengua materna Maltés Información profesionalOcupación Cantante y modelo.Años activa desde 2006Seudónimo «El Volcán Mediterráneo»Géneros Pop, danceInstrumento VozSitio web Página oficial[editar datos en Wikidata] Margaret Camilleri conocida como Morena,[1] nacida en el año de 1984,[...

Circuit simulation software LTspiceOriginal author(s)Mike Engelhardt[1]Developer(s)Linear Technology,[1] Analog Devices[2]Initial releaseOctober 1999;24 years ago (1999-10)[1]Stable release17.1.15[3] / October 2, 2023 (2023-10-02)[3] Operating systemWindows 7, 8, 8.1, 10, macOS 10.10+PlatformIA-32, x86-64SizeWindows (60 MB), MacOS (30 MB)Available inEnglishTypeElectronic design automationLicenseFreeware[4 ...

Cet article est une ébauche concernant le Burundi. Vous pouvez partager vos connaissances en l’améliorant (comment ?) selon les recommandations des projets correspondants. 2007 au Burundi - 2008 au Burundi - 2009 au Burundi - 2010 au Burundi - 2011 au Burundi 2007 par pays en Afrique - 2008 par pays en Afrique - 2009 par pays en Afrique - 2010 par pays en Afrique - 2011 par pays en Afrique] Chronologies Données clés 2006 2007 2008 2009 2010 2011 2012Décennies :197...

[1]هذه مقالة غير مراجعة. ينبغي أن يزال هذا القالب بعد أن يراجعها محرر؛ إذا لزم الأمر فيجب أن توسم المقالة بقوالب الصيانة المناسبة. يمكن أيضاً تقديم طلب لمراجعة المقالة في الصفحة المخصصة لذلك. (أبريل 2021) هذه المقالة يتيمة إذ تصل إليها مقالات أخرى قليلة جدًا. فضلًا، ساعد بإ�...
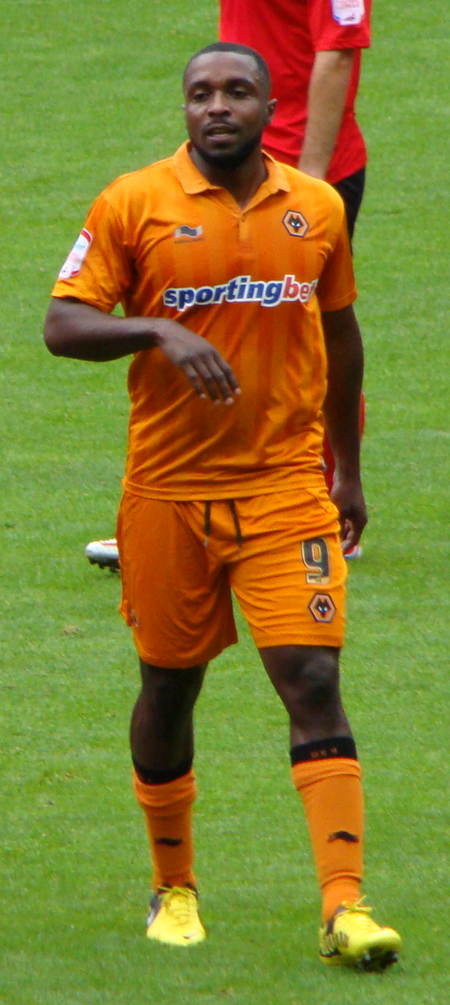
Sylvan Ebanks-Blake Ebanks-Blake bermain untuk Wolverhampton Wanderers pada 2012Informasi pribadiNama lengkap Sylvan Agustus Ebanks-Blake[1]Tanggal lahir 29 Maret 1986 (umur 37)Tempat lahir Cambridge, InggrisTinggi 1,78 m (5 ft 10 in)[2]Posisi bermain PenyerangKarier junior1999–2002 Cambridge United2002–2004 Manchester UnitedKarier senior*Tahun Tim Tampil (Gol)2004–2006 Manchester United 0 (0)2006 → Royal Antwerp (pinjaman) 9 (4)2006–2008 Plymout...

Paghimo ni bot Lsjbot. Anthurium holquinianum Siyentipikinhong Pagklasipikar Kaginharian: Plantae Kabahig: Tracheophyta Kahutong: Liliopsida Kahanay: Alismatales Kabanay: Araceae Kahenera: 'Anthurium' Espesye: ''Anthurium holquinianum'' Siyentipikinhong Ngalan Anthurium holquinianumCroat & D.C.Bay Kaliwatan sa tanom nga bulak ang Anthurium holquinianum.[1] Una ning gihulagway ni Thomas Bernard Croat ug D.C.Bay.[2] Ang Anthurium holquinianum sakop sa kahenera nga Anthurium,...